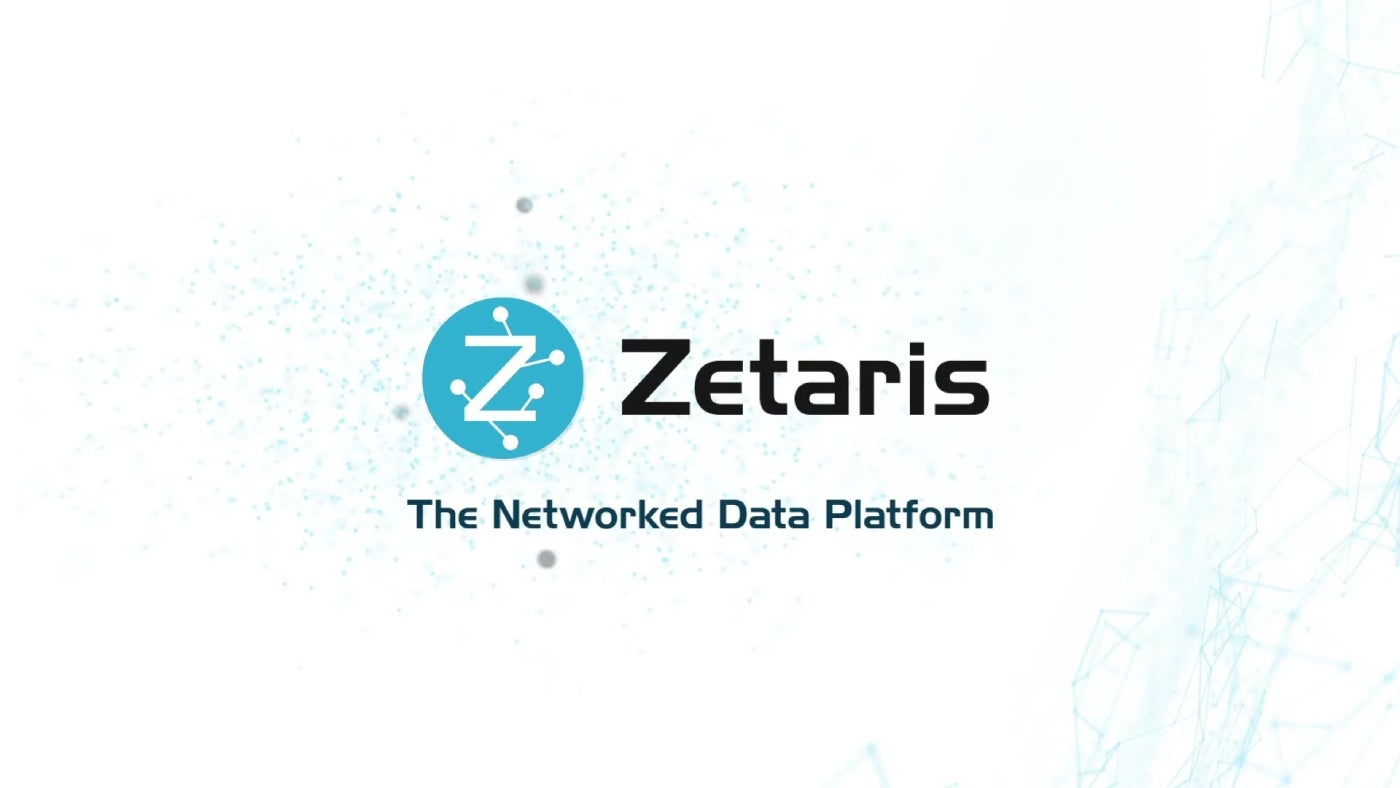
Australian organisations have tried exhausting to convey knowledge collectively in latest many years. They’ve moved from knowledge marts, which contained data particular to enterprise models, to knowledge warehouses, knowledge lakes and now lakehouses, which comprise structured and unstructured knowledge.
Nonetheless, the idea of the federated lakehouse may now be successful the day. Taking off within the U.S., Vinay Samuel, CEO of information analytics virtualisation agency Zetaris, tells TechRepublic actuality is forcing organisations to construct roads to knowledge the place it resides quite than try and centralise it.
Zetaris founders realised knowledge may by no means be totally centralised
TR: What made you resolve to start out Zetaris again in 2013?
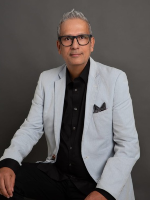
Samuel: Zetaris got here out of a protracted journey I had been on in knowledge warehousing — what they used to name the massive database world. That is again within the Nineties, when Australian banks, telcos, retailers and governments would gather knowledge principally for resolution assist and reporting to do (enterprise intelligence) sort of issues.
PREMIUM: Key options companies ought to contemplate when selecting a cloud knowledge warehouse.
The one factor we discovered was: Clients had been frequently looking for the following finest knowledge platform. They frequently began initiatives, tried to affix all their knowledge, convey it collectively. And we requested ourselves, “Why is it that the client may by no means get to what they had been making an attempt to attain?” — which was actually a single view of all their knowledge in a single place.
The reply was: It was simply inconceivable. It was too exhausting to convey all the info collectively within the time that will make sense for the enterprise resolution that was needing to be resolved.
TR: What was your strategy to fixing this knowledge centralisation drawback?
Samuel: After we began the corporate, we stated, “What if we problem the premise that, to do analytics on knowledge or reporting in your day-to-day, you need to convey it collectively?”
We stated, “Let’s create a system the place you didn’t need to convey knowledge collectively. You might go away it in place, wherever it’s, and analyse it the place it was created, quite than transfer it into, you realize, the following finest knowledge platform.”
That’s how the corporate began, and fairly frankly, that was an enormous problem. You wanted large compute. It wanted a brand new sort of software program; what we now name analytical knowledge virtualisation software program. It took us a very long time to iterate on that drawback and land on a mannequin that labored and would take over from the place organisations are right this moment or had been yesterday.
TR: That should seem to be an awesome resolution now AI is actually taking off.
Samuel: I suppose we landed on the thought pretty early in 2013, and that was factor as a result of it was going to take us 5 to 6 or seven years to really iterate on that concept and construct the question optimizer functionality that permits it.
This entire shift in direction of real-time analytics, in direction of real-time AI, or generative AI, has meant that what we do has now turn into essential, not only a good to have thought that might save an organisation some cash.
The final 18 months or so have been unbelievable. At present, organisations are shifting in direction of bringing generative AI or the sort of processing we see with Chat GPT on high of their enterprise knowledge. To try this, you completely want to have the ability to deal with knowledge in every single place throughout your knowledge lake. You don’t have the time or the posh to convey knowledge collectively to scrub it, to order it and to do all of the issues you need to do to create a single database view of your knowledge.
AI progress means enterprises need to entry all knowledge in actual time
TR: So has the Zetaris worth proposition modified over time?
Samuel: Within the early years, the worth proposition was predominantly about value financial savings. You recognize, when you don’t have to maneuver your knowledge to a central knowledge warehouse or transfer all of it to a cloud knowledge warehouse, you’ll prevent some huge cash, proper? That was our price proposition. We may prevent some huge cash and allow you to do the identical queries and go away the info the place it’s. That additionally has some inherent safety advantages. As a result of when you don’t transfer knowledge, it’s safer.
Whereas we had been undoubtedly doing properly with that worth proposition, it wasn’t sufficient to get individuals to only leap up and say, “I completely want this.” With the shift to AI, now not are you able to watch for the info or settle for you’ll solely do your analytics on the a part of your dataset that’s within the knowledge warehouse or knowledge lake.
The expectation is: Your AI can see all of your knowledge, and it’s in a form able to be analysed from a knowledge high quality standpoint and a governance standpoint.
TR: What would you say your distinctive promoting proposition is right this moment?
Samuel: We allow prospects to run analytics on all the info, irrespective of the place it’s, and supply them with a single level of entry on the info in a approach that it’s protected to take action.
It’s not simply with the ability to present a person with entry to all the info within the cloud and throughout the info centre. It’s additionally about being cognizant of who the person is, what the use case is, and whether or not it’s acceptable from a privateness, governance and regulatory standpoint and managing and governing that entry.
SEE: Australian organisations are struggling to stability personalisation and privateness.
We have now additionally turn into a knowledge server for AI. We allow organisations to create the content material retailer for AI purposes.
There’s a factor referred to as retrieval-augmented era, which lets you increase the era of (a big language mannequin) reply to a immediate together with your personal knowledge. And to do this, you’ve bought to verify the info is prepared and it’s accessible — it’s in the best format, it has the best knowledge high quality.
We’re that utility that prepares the info for AI.
Information readiness is a key barrier to profitable AI deployments
TR: What issues are you seeing organisations having with AI?
Samuel: We’re seeing a whole lot of firms eager to develop an AI functionality. We discover the primary barrier they hit just isn’t the problem of getting a bunch of information scientists collectively or discovering that incredible algorithm that may do mortgage lending or predict utilization on a community, relying on the business the client is in.
As a substitute, it’s to do with knowledge readiness and knowledge entry. As a result of if you wish to do ChatGPT-style processing in your personal knowledge, typically the enterprise knowledge simply isn’t prepared. It’s not in the best form. It’s somewhere else, with completely different ranges of high quality.
And so the very first thing they discover is they really have a knowledge administration problem.
TR: Are you seeing an issue with hallucinations in enterprise AI fashions?
Samuel: One of many causes we exist is to negate hallucination. We apply reasoning fashions, and we apply varied methods and filters, to verify the responses which are being given by a personal LLM earlier than they’re consumed. And what which means is that it’s often checked towards the content material retailer that’s being created from the client’s personal knowledge.
So as an example, a easy hallucination may very well be {that a} buyer in a financial institution, who’s in a decrease wealth section, is obtainable a large mortgage. That may very well be a hallucination. That simply merely received’t occur if our tech is used on high of the LLM as a result of our tech is speaking to the true knowledge and is analysing that buyer’s wealth profile and making use of all of the regulatory and compliance guidelines.
TR: Are there another widespread knowledge challenges you’re seeing?
Samuel: A standard problem is mixing various kinds of knowledge to reply a enterprise query.
As an illustration, giant banks are accumulating a whole lot of object knowledge — footage, sound, machine knowledge. They’re making an attempt to work out easy methods to use that in live performance with conventional type of transaction financial institution assertion knowledge.
It’s fairly a problem to work out the way you convey each these structured and unstructured knowledge varieties collectively in a approach that may improve the reply to a enterprise query.
For instance, a enterprise query is likely to be, “What’s the proper or subsequent finest wealth administration product for this buyer?” That’s given my understanding of comparable prospects over the past 20 years and all the opposite data I’ve from the web and in my community on this buyer.
The problem of bringing structured and unstructured knowledge collectively right into a deep analytics query is a problem of accessing the info somewhere else and in several shapes.
Clients utilizing AI to suggest investments, heal networks
TR: Do you’ve examples of the way you assist prospects make use of information and AI?
Samuel: We have now been working with one giant wealth administration group in Australia, the place we’re used to jot down their suggestion studies. Up to now, an precise wealth supervisor must spend weeks, if not months, analysing lots of, if not 1000’s, of PDFs, picture information, transaction knowledge and BI studies to give you the best portfolio suggestion.
At present, it’s occurring in seconds. All of that’s occurring, and it’s not a pie chart or a pattern, it’s a written suggestion. That is the mixing of AI with automated data administration.
And that’s what we do; we mix AI with automated data administration to unravel that drawback of what’s the following finest wealth administration product for a buyer.
Within the telecommunications sector, we’re serving to to automate community administration. An enormous drawback telcos have is when some a part of their infrastructure fails. They’ve about 5 – 6 completely different potential explanation why a tower is failing or their gadgets failing.
With AI, we are able to rapidly shut in on what the issue is to allow the self-healing strategy of that community.
TR: What is especially attention-grabbing within the generative AI work you’re doing?
Samuel: What is actually wonderful for me is that, due to the way in which we’re doing it, our know-how now permits regular human beings who don’t know easy methods to code to speak to the info. With generative AI on high of our knowledge platform, we’re in a position to categorical queries utilizing pure language quite than code, and that actually opens up the worth of the info to the enterprise.
Historically, there was a technical hole between a enterprise individual and the info. Should you didn’t know easy methods to code and when you didn’t know easy methods to write SQL rather well, you couldn’t actually ask the enterprise questions you wished to ask. You’d need to get some assist. Then, there was a translation situation between the people who find themselves making an attempt to assist and the enterprise practitioner.
Properly, that’s gone away now. A sensible enterprise practitioner, utilizing generative AI on high of personal knowledge, now has that functionality to speak on to the info and never fear about coding. That actually opens up the potential for some actually attention-grabbing use instances in each business.
Australia follows America in seeing worth of federated lakehouse
TR: Zetaris was born in Australia. Are your prospects all Australian?
Samuel: During the last 18 months, we’ve had fairly a robust give attention to the American market, particularly within the industries which are shifting quickest, like healthcare, banks, telcos retailers, producers, and we’re getting some authorities curiosity as properly. We now have about 40 individuals.
Australia is the hub, however we’re unfold throughout the Philippines and India and have a small footprint in America.
The use instances are attention-grabbing and are to do with analysing the info wherever with generative AI. As an illustration, we’re now serving to a big hospital group do triage. When a affected person comes into the group, they’re utilizing generative AI to in a short time make selections on whether or not somebody’s chest ache is a panic assault or whether or not it’s really a coronary heart assault or no matter it’s.
TR: Is Australia coming nearer to adopting the thought of the federated lakehouse?
Samuel: The (Australian) market tends to comply with the American market. It’s often a few yr behind.
We see it loud and clear in America {that a} lakehouse doesn’t need to imply centralised; there’s an acceptance of the concept that you’ll have a few of your knowledge within the lakehouse, however then, you’ll have satellites of information wherever else. And that’s been pushed by actuality, together with firms having a number of footprints throughout the cloud; it’s common for many enterprises to have two or three cloud distributors supporting them and a big knowledge centre footprint.
That’s a pattern in America, and we’re beginning to see shoots of that in Australia.
Change won’t enable knowledge consolidation in a single location
TR: So the thought of centralising organisational knowledge remains to be inconceivable?
Samuel: The notion of bringing it collectively and consolidating it in a single knowledge warehouse or one cloud — I consider, and we nonetheless consider — is definitely inconceivable.
We noticed the problem banks, telcos, retailers and governments confronted after we began with resolution assist and knowledge administration, and fairly frankly, the mess knowledge was and nonetheless is in giant enterprises. As a result of knowledge is available in completely different shapes, ranges of high quality, ranges of governance and from a myriad of purposes from the info centre to the cloud.
Significantly now, while you have a look at the velocity of enterprise and the quantity of change we’re dealing with, purposes that generate knowledge are frequently being found and introduced into organisations. The quantity of change doesn’t enable for that single consolidation of information.